Diagnostic Model Using Machine Learning to Detect Acute Interstitial Nephritis Shows Promise
Johns Hopkins Medicine and Yale University have collaborated on a diagnostic model to detect acute interstitial nephritis (AIN) in patients.
AIN is “a common cause of acute kidney injury, or AKI, marked by swelling and inflammation of certain kidney tissues, and generally linked to use of medicines such as steroids, proton pump inhibitors and antibiotics.” AKI affects “one in five hospitalized patients,” and it can be difficult to differentiate AIN from other causes of AKI. Over 90% of patients with AIN have no signs or symptoms, and delayed or missed diagnoses could “potentially lead to permanent kidney damage.”
In this new study, researchers developed a diagnostic model to predict AIN by “using a machine-learning selection technique called least absolute shrinkage and selection operator (LASSO). The laboratory tests using the LASSO feature included serum creatinine, blood urea nitrogen (BUN), urine protein, and the density of urine compared to water, which is known as a specific gravity test.”
The study consisted of two patient cohorts. Both had members who had “previously undergone kidney biopsy procedures,” excluding certain patients. 1,982 patients were examined in total and “a combined 22% of them were found to have been diagnosed with AIN. In both cohorts, those with AIN were more likely to be hospitalized, and had higher serum creatinine and blood urea nitrogen to creatinine ratio. While the diagnostic model was able to improve the accuracy of AIN diagnosis for clinicians to 77%, there were noted differences in the prevalence of AIN in both patient cohorts.”
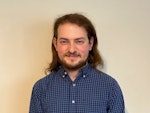
Matt MacKenzie | Associate Editor
Matt is Associate Editor for Healthcare Purchasing News.