Study Finds Large Language Models Are Less Accurate Analyzing Patient-Written Summaries
NIH researchers have discovered that artificial intelligence tools are “significantly less accurate when analyzing summaries written by patients about their own health” compared to when given “textbook-like descriptions of genetic diseases.”
Researchers tested 10 different large language models by designing questions about 63 different genetic conditions, some well-known and some much rarer. They aimed to “capture some of the most common possibly symptoms.”
Initially, the large language models “ranged widely in their ability to point to the correct genetic diagnosis, with initial accuracies between 21% and 90%.” Their success “generally corresponded with their size, meaning the amount of data the models were trained on.”
The researchers “optimized and tested the models in various ways,” including by substituting more common language in place of medical terms. The models’ accuracy overall “decreased when medical descriptions were removed,” but 7 out of 10 of the models were “still more accurate than Google searches when using common language.”
Researchers then asked patients from the NIH Clinical Center to “provide short write-ups about their own genetic conditions and symptoms. These descriptions ranged from a sentence to a few paragraphs and were also more variable in style and content compared to the textbook-like questions.” The best-performing model made accurate diagnoses only 21% of the time when presented with these write-ups, and “many models performed much worse, even as low as 1% accurate.”
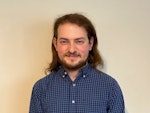
Matt MacKenzie | Associate Editor
Matt is Associate Editor for Healthcare Purchasing News.