AI: Opportunities, threats meet, tease healthcare supply chain
To adapt a refrain from the cartoon philosopher Homer Simpson, “AI – the cause of and solution to ALL of supply chain’s problems.”
For some (e.g., entrepreneurs, innovators and opportunists, etc.), AI represents shortcuts and workarounds to be more efficient at work and either enjoy more recreational time or focus on other expedient tasks courtesy of the additional time.
For others (e.g., conspiracy theorists, cynics and skeptics, etc.), artificial intelligence (AI) calls to mind robots besmirching humans through deep fake audio, video and text or even replacing humans for jobs and revenue and taking over the world.
Still for others (e.g., forward-thinking dreamers and open-minded schemers), AI represents the first-generation leap to Star Trek-like automated decision support with automatic universal translators (no need to take language courses in high school and college to graduate!) that should accurately second-guess even the second-guessers on a global scale.
Recently, the U.S. Food and Drug Administration jumped into the frenzy by creating a new Digital Health Advisory Committee “to explore the complex, scientific and technical issues related to digital health technologies, such as artificial intelligence.”
At least one study posits that AI likely will reach a half-a-trillion-dollar industry by 2027, giving additional meaning to “generative” AI. That’s within three years and represents a faster market stickiness (in terms of prospective adoption and implementation) and predictive revenue trajectory than the internet and online exchanges, internet-of-things (IoT) and blockchain achieved during their hype cycles.
But beyond the hype, pomp and circumstance, does AI amount to anything more than a decision-support tool that requires training for the end user as well as the application itself? Is it a generative relative of blockchain? A veritable electronic Swiss Army Knife for healthcare supply chain? A “shadow” database/virtual professional assistant not unlike Amazon’s Alexa, Apple’s Siri, Google’s Assistant with Bard or Microsoft’s Cortana?
Healthcare Purchasing News reached out to numerous corporate executives on the supplier and provider segments of the industry to gauge their anticipation, application and prognostication of AI as it relates to supply chain operations. The wide-ranging exploration spanned the promise of process effectiveness and efficiency to the precariousness of potential abuse, control and cybersecurity dangers.
Optimists foresee much promise; pessimists highlight areas of concern that if left unchecked could have considerable repercussions. Overall, optimism, which is shared here and on HPN Online, seems to surpass pessimism, which can be found on HPN Online.
Several experts salute the promise that AI brings to supply chain, particularly around data collection and number crunching.
“Artificial intelligence will be able to make use of large amounts of data to make accurate predictions around usage and supply availability,” said Jack Koczela, director, Sourcing & Transformation, Supply Chain, Froedtert Health. “It can also comb through data from different sources, such as clinical systems and business systems, to create insights that we have not been able to process at scale in the past. For me, this is one of the most exciting applications of artificial intelligence. As we move into a clinically integrated supply chain, we have many small, manual models that indicate we could see significant improvement if we could just get through all the data that is available at our fingertips.”
Archie Mayani, chief product officer, GHX, marvels at the past decade of development and growth of interest in AI, particularly for supply chain applications.
“Ten years ago, the healthcare industry was just beginning to talk about AI,” she recalled. “Now, it is beginning to transform healthcare and, more specifically, the supply chain. As the industry continues to use generative AI to sort and manage the influx of data, supply chain management processes will become more predictive, effective and efficient. If used correctly, AI has the potential to refocus healthcare teams on higher-value work such as supply chain resiliency and aid in future crisis mitigation, waste reduction, and cost-containment efforts that will help hospitals reinvest funds into patient care. In the era of smart supply chains, AI is the prescription for optimizing, not just organizing.”
But another supply chain expert offers a profound observation seasoned with a pinch of irony that suits today’s workforce.
“AI can help us make better use of scarce labor and rid ourselves of repetitive low cognitive work,” noted Joe Dudas, division chair, Supply Chain Innovation and Strategy, Mayo Clinic. “It can change our jobs to be more human.”
Accentuate the obvious
The mere notion of AI motivates many experts to list a bevy of obvious applications that can benefit supply chain operations. A dozen experts share their insights with HPN that at times converge on common themes.
“Proper inventory management is a core competency for efficient supply chain management and an area where AI applications will continue to emerge for the foreseeable future,” assured Vasco Kollokian, director, Innovation and AI, Tecsys. “By analyzing historical supplies consumption data and using machine learning for demand forecasting, AI can predict accurate levels of inventory to ensure optimal stock levels.
“Once optimal levels have been established for a particular healthcare provider network, maintaining suitable levels of inventory through location-specific replenishment becomes another clear application for AI,” he continued. “Leveraging dynamic real-time inventory visibility through IoT-based technologies, AI-driven processes can optimize the minimum and maximum inventory counts and automatically replenish to match various hospitals’ consumption-driven needs for specific locations, such as operating rooms, catheter labs, nursing floors and in-hospital pharmacies.”
Kollokian further believes that AI will interface or integrate with materials management information systems (MMIS), enterprise resource planning (ERP) systems, item masters and chargemasters, regardless of brand. “ERPs typically have control over item masters,” he indicated. “If visibility into fine-grained location-specific consumption and replenishment information is provided by other, more specialized, vendors, then integration is often necessary.”
The root of such connectivity varies between built-in product programming, “bolt-on” software application or online download.
“Usually, integration APIs are provided by vendors to accommodate information exchange among disparate supply chain management solution systems,” Kollokian explained. “For example, order quantities in ERPs typically are at the ‘pallet’ level, yet Automated Dispensing Cabinets (ADC) replenish at ‘each’ or ‘box’ level. Such system disparity often results in fragmented information fabric, requiring a ‘bolt-on’ approach. Whether vendors agree to provide such integration (usually considered competitive) is an orthogonal matter.”
Demand planning rules
The global pandemic elevated predictive demand planning to the forefront of “must-have” capabilities on automated wish lists, and Kollokian agrees that it’s ripe for AI.
“While some demand spikes are quite apparent, like seasonal demand for flu vaccines, others are less so,” he indicated. “For example, states not mandating motorcycle helmets are likely to see increased head trauma cases resulting from motorcycle accidents, prompting the need for proactive prior stock-up. AI can detect epidemiological healthcare patterns for less apparent trends without reliance on tribal knowledge.”
John Freund, president and CEO, Jump Technologies, zeroes in on demand forecasting as the most obvious use. “AI algorithms can analyze historical sales data, patient demographics and other relevant factors to predict future demand more precisely than traditional methods,” he told HPN. “This can help healthcare providers optimize inventory levels, reducing costs and improving access to essential medications and supplies.”
Connectivity throughout multiple systems for supply chain is essential, according to Freund.
“The demand forecasting engine needs consumption information so the inventory management tool would be needed either from the ERP system or third-party system like JumpStock,” he indicated. “It also needs vendor performance information at the product level, so it needs receipt data either from the ERP system or third-party system. It needs census data, so it needs data from the EHR. There may also be a variety of other third-party data sets that are needed to do this properly. An AI-based demand forecasting engine will need data from a variety of sources.”
Expanding supply chain automation and optimization beyond current capabilities is another valuable contribution, Freund posits.
“AI can automate various tasks throughout the healthcare supply chain, from procurement and ordering to warehousing and distribution,” he said. “For example, AI-powered chatbots can handle routine customer inquiries, while robotic process automation (RPA) bots can automate repetitive tasks such as data entry and order processing. This can free up human workers to focus on more complex tasks, improve efficiency, and reduce errors.”
But AI doesn’t necessarily duplicate other pre-existing automated tasks, he insists.
“Today in many hospitals requisitions flow down to purchasing, who in turn place those orders with vendors, in some cases considering multiple sources for a given product,” Freund explained. “AI can automate that entire process, taking the human element out of it. Think of the number of calls to purchasing looking for order status. AI chatbots can analyze data on product defects and customer complaints to identify potential quality issues. Chatbots can [help] hospitals with regulatory requirements by tracking and reporting recall levels. There is a wide variety of ways that AI can work here.”
Arnold Chazal, CEO, VUEMED Inc., remains solidly behind AI as creating more accurate predictive models for supply re-ordering and replenishment and balance for inventory management. “AI can help ensure that proper orders with the adequate quantities are placed in time to support the clinical work regardless of the cyclical or seasonal variations and take into account the greater supply chain challenges such as backorders and recalls,” he noted.
“AI can optimize even more dynamically the on-hand inventory to meet the needs of each individual clinician by avoiding stock-outs while at the same time avoiding over-stocking, Chazal continued. “AI can do this by learning to predict what each clinician will actually need and use for each type of procedure, taking into account the specifics of anatomy and disease stage of patients to be treated based on their medical records. This application will definitely be helpful for addressing the case cart and preference cards disconnects with the clinical requirements once the patient is being treated, thus ensuring the preparation of a case cart that is more adapted to the physician’s practice and habits and the patient being treated. It will advance customized care to benefit both the patient and the clinicians.”
AI can enable supply demand forecasting for providers and suppliers alike, observes Tom Redding, senior managing director, Healthcare, St. Onge Co.
“Unfortunately, the healthcare supply chain primarily has a transactional relationship with their health system,” Redding lamented. “There is a significant potential to gain insights from transactional history to inform future purchasing decisions. Additionally, it may inform any contract negotiations with their suppliers to ensure they can meet the organization’s total demand requirements and the anticipated demand variation. Health systems are stuck in the allocation model, which drives them to seek other product options during peak periods.”
AI can assist supply chain operations within clinics, surgery centers and other non-acute facilities, too, Redding stresses.
“With more ‘low-demand’ non-acute facilities coming online in the coming years, there’s a need to continually monitor demand and adjust the available units of measure,” he noted. “There are opportunities to right-size the required units of measure. Manufacturers spend a considerable amount of time trying to determine the packaging size to optimize their costs but that doesn’t mean it meets the needs of their customers.”
GHX’s Mayani sees AI improving demand planning and forecasting overall based on data access. “AI can analyze historical and current inventory utilization data to help a hospital more accurately predict the demand for various medical supplies and equipment,” she said. “With the help of AI, high-performing hospital supply chains have an opportunity to reduce supply expenses as well as ensure the right product is available at the right time for the right procedure to support better clinical outcomes.”
Yet AI makes sense for improving clinical inventory management at the point-of-use level as well, according to Mayani. “As healthcare decision-makers consider inventory levels and their related inventory systems, inaccuracy at the point-of-use can create significant operational hurdles when trying to either reduce costs or maintain high service levels. AI models can help drive supply capture at the point-of-use and automate and streamline these workflows with seamless and interoperable data with electronic medical records.”
St. Onge’s Redding foresees AI driving inventory management optimization. “In most cases today, the process to manage clinical inventories is still a predominately manual and an arduous process,” he noted. “AI can provide a real-time monitoring and provide adjustments to how much inventory is needed and make decisions on when to order supplies to eliminate guess work.”
If anything, AI can help the healthcare industry speed up demand planning communications, according to Josh Wolfe, senior vice president, Inventory Management, Medline. “In an industry where data sharing and integration between trading partners has lagged, AI could help provide more accurate demand signals, resulting in fewer supply disruptions,” he noted.
Bruce Lieberthal, vice president and Chief Innovation Officer, Henry Schein, agrees on demand forecasting’s inherent benefits. “AI can be used to consider the myriad of factors affecting demand to help create forecasting models that are more accurate and cost-effective,” he said. “This is important so that healthcare companies can reduce stockouts or over-ordering.”
Maintaining equipment
Predictive maintenance for medical equipment is another function that AI could manage, according to JumpTech’s Freund. “Medical equipment is expensive and critical for patient care,” he indicated. “AI-powered predictive maintenance systems can analyze sensor data from medical devices to identify potential malfunctions before they occur. This proactive approach can help prevent costly downtime, extend the lifespan of equipment, and ensure that critical devices are always available for patient care.”
This extends a step further than the real-time maintenance programs offered by several original equipment manufacturers that automatically alert the OEM when something goes wrong onsite at the provider facility to minimize operational downtime.
“AI algorithms can go deeper than purely maintenance data,” Freund assured. “For example, AI could study sensor data from machines used to coat the joints with a biocompatible material. The algorithms can detect defects in the coating, such as air bubbles or uneven thickness. AI can be used to optimize the coating process by adjusting the machines' speed, improving the joint's quality or reducing recalls.”
For James Kollai, senior director, Client Solutions, PartsSource, AI reaches into the parts and inventory management aspects of equipment maintenance. “AI could optimize inventory management by predicting which parts are likely to fail and when,” he said. “This would then help clinical engineering and healthcare technology management teams ensure that the right components are available when needed without excessive stockpiling.”
PartsSource strives to integrate AI in critical decision support for dual and digital marketplace of providers and suppliers, according to Kollai. “One of the more obvious applications where we have used AI is through lead time predictions,” he noted. “In recent months, we have established a process leveraging our industry leading supply chain and transactional data sets along with a proprietary AI to predict lead times for a large portion of items purchased from our online marketplace. Approximately 90% or more vendors do not provide inventory feeds with accurate shipping information, which is crucial to customers who need parts to repair their medical equipment. Our AI can predict shipping times based on data from previous purchases by customers from the supplier to accurately fill in those inventory gaps.
“Additionally, PartsSource uses AI to help inform our Guaranteed Stock offering for our customers,” Kollai added. “Our teams are currently working on integrating AI for forward stocking, also called local stocking, options to minimize equipment downtime.”
Accentuate the alternatives
In addition to demand planning, AI can help manage clinically acceptable alternative products and sourcing, which attained a fever pitch during the COVID-19 pandemic.
“Given the new normal of supply disruptions, the ability to efficiently and accurately recommend clinically viable alternate products is a necessity in today’s healthcare supply chain,” indicated Medline’s Wolfe. “AI provides some exciting opportunities to meet that need.”
GHX’s Mayani contends AI can compile a list of substitutes to help avoid backorders and shortages, effectively serving as an extension of demand planning. “AI has the ability to offer more efficient and effective ways to manage supply chain disruptions and shortages,” she indicated. “Machine learning (ML) and AI algorithms can easily analyze historical data and market trends to predict potential backorders and make recommendations on product alternatives and substitutes based on evidence-based clinical outcomes. This not only helps limit overstocking and reduce waste, but also helps drive value-based care upstream and across the continuum of care.”
Carl Natenstedt, CEO, Z5 Inventory, concurs. “AI can be used to automate the ‘alternate sourcing scurry’ that occurs during backorders and product shortages,” he said. “AI can learn from past product shortage resolutions to mimic the human sourcing function and identify alternate sources of the same or similar product and place emergency orders.”
Evaluating supplier service
AI can assist in making considerable progress with supplier performance measures and programs, St. Onge’s Redding suggests. “Health systems will gravitate to AI to inform their decision-making process on which suppliers will get their business in the future,” he said. “Health systems can’t simply look at the cost of the product only. They will need to take into consideration the total cost of the relationship. If the health system takes into the consideration all their internal time and effort to resolve issues with a supplier, substituting supplies from a different supplier potentially at a higher cost, expediting freight costs and many other factors, the supplier relationship may not be worth all the effort.”
Product access and availability are the triggers, Redding insists.
“Every health system will share without pause that product availability is their biggest challenge daily; it was a challenge prior to the pandemic, and it has accelerated since the pandemic,” he noted. “Health systems will need leverage AI to continually monitor supplier performance and gain insights into the health of their suppliers. Creating a reliable, efficient and resilient supply chain starts with collaboratively sharing data/information between the health system and suppliers to ensure there is end-to-end visibility and each party can inform their internal decisions. Too often, we see reluctance to share data/information because there is concern that the other party may take advantage of the situation, either from a cost savings point of view and/or shifting away from the supplier because of lack of internal performance.”
Homing in on the granular
Demand management and monitoring warehouse performance top the list for George S. Godfrey, chief supply chain officer and corporate vice president, Financial Shared Services, Baptist Health South Florida, but he takes a cue from Amazon Prime, FedEx and UPS and points to delivery calendars for inbound and outbound shipments to manage “real-time scheduling that adjusts to traffic delays, urgency of goods, etc.”
Z5 Inventory’s Natenstedt sees benefits to automated and constant PAR area optimization and physician preference card management and maintenance from AI deployment, too.
“AI can learn from each replenishment assessment the consumption patterns of each specific PAR area and apply intelligent adjustments on a near-daily basis to streamline and reduce investment in inventory,” he indicated. “AI can learn from past case records and post-case waste and returned product assessments to constantly update and adjust the items on preference cards and their classifications to reduce product waste in the OR.”
Editor’s Note: For additional exclusive coverage of AI’s not-so-obvious uses, potential dangers and specific areas where AI is and will make clinical, financial and operational inroads relating to supply chain, visit HPN Online. Back in November 2023, HPN conducted an AI Virtual Forum, “Can AI Ease Current Healthcare Burdens?” To view it, click here: https://endeavor.swoogo.com/2023HPNVirtualForum/todays-landscape.
Pulling back the curtain on second-thought AI benefits for healthcare supply chain
What if AI can help with or manage this …
Is the time ripe for a “SCarlet?”
Counterfeit detection, managing risk
Tracking equipment performance
Fine-tunning supply chain operations
- Expiration Date Prediction in Pharmaceutical Supply Chain Management: “AI can be leveraged to predict the remaining shelf life of pharmaceuticals, aiding in the efficient management of inventory and minimizing wastage due to expired products,” she said. “By analyzing factors such as storage conditions, product characteristics and historical usage patterns, AI algorithms can predict expiration dates more accurately than traditional static shelf-life assignments. This can help reduce waste by eliminating the discarding of still-usable items.”
- Carbon Footprint Optimization: “AI can help optimize the carbon footprint of the healthcare supply chain by providing insights into the environmental impact of transportation, packaging and procurement decisions, Mayani continued. “AI algorithms can analyze data related to transportation routes, packaging materials and supplier locations to suggest environmentally friendly alternatives including the last mile. This not only aligns with sustainability goals but can lead to cost savings by identifying more efficient and eco-friendly supply chain practices.”
- Clinical Trial Material Forecasting: “AI can be applied to forecasting demand for materials used in clinical trials, optimizing the supply chain specifically for trial-related products,” she indicated. “Clinical trials often involve unique and specialized materials, and predicting the demand for these items can be challenging. AI algorithms that leverage historical trial data, patient enrollment patterns and external factors like patient demographics, disease prevalence and incidence rates, economic conditions, and geopolitical events, can provide more accurate forecasts. This helps ensure the necessary materials are available when needed, preventing delays in trial timelines and improving overall trial efficiency.”
Provider practicality
- AI can be used to manage the resolution process associated with invoice match exceptions.
- AI can be used to assist the contract activation process after you start the contract renewal proceedings.
- AI can be used to monitor each nurse station supply cabinet and adjust min/max inventory levels based on specific demand patterns for a particular nurse station, he adds.
How much clear and present danger lurks amid AI fog?
Obvious dangers
- Data and privacy security concerns: “Healthcare data, including supply chain information and data, is highly sensitive. Ensuring the privacy and security of this data is crucial. AI systems must comply with strict regulations such as HIPAA, GDPR and other regional laws that govern data privacy and security.”
- Interoperability and data standardization: “Healthcare data often resides in diverse systems and silos that may not easily communicate with each other. Integrating AI solutions with existing infrastructure and ensuring data standardization across platforms can be particularly challenging, leading to interoperability issues.”
- Data quality and availability: “AI algorithms rely on high-quality, comprehensive datasets. In healthcare supply chain management, obtaining accurate and up-to-date data can be challenging due to variations in data sources, data entry errors and differing data formats,” she indicated.
New role brewing, emerging?
Not-so-obvious dangers
AI’s meaningful contributions to key supply chain issues
Healthcare Purchasing News concentrated on several key areas – departments and functions – where artificial intelligence can play a pivotal role in reshaping and improving practices and processes and asked provider- and supplier-based corporate supply chain executives what they reasonably might expect. Here’s what they shared.
1. To cleanse their data routinely and/or seek out and/or use only authenticated, trustworthy, validated data and potentially obviate the need for or replace data-cleansing services and software
“AI algorithms can identify and remove duplicate records, incomplete data and erroneous entries from hospital databases. This can help to improve the quality and accuracy of data, making it easier for clinicians and researchers to access and analyze reliable information. AI can be used to identify unusual patterns or outliers in data, which may indicate potential errors or fraud. This can help to proactively address data quality issues before they cause problems. AI can be used to monitor data streams in real time, identifying and flagging potential issues as they occur. This can help to prevent data quality problems from escalating and disrupting workflows.” On replacing data cleansing services and software: “Yes.”
--John Freund, president and CEO, Jump Technologies
“Having inconsistent item master data with missing or incorrect Universal Device Identifiers (UDI) like GTINs will cause all sorts of supply chain hurdles. No GTIN means that items cannot be scanned, ordered in the right quantities, received or billed. Using a variety of techniques such as fuzzy searches and semantic associations, abbreviation disambiguation, AI can leverage trusted data sources to continuously resolve and cleanse UDI’s in item masters, rectifying their package multipliers so proper items and quantities can be ordered.” On replacing data cleansing services and software: “Progressively yes, although they themselves might leverage AI techniques under the covers.”
--Vasco Kollokian, director, Innovation and AI, Tecsys
“While some providers may use artificial intelligence to cleanse and/or update their data, many providers will probably look to third parties to provide this service for them. It would be my expectation that any third party providing this service would have an AI-enabled solution. This should allow them to scale their solutions to the size of modern item masters and SC data needs. Once, a provider’s item master only contained 10-20,000 items. Now these files have grown to 500,000+ lines long. This amount of information is impossible for a human to cleanse. But using AI rules it should be relatively straightforward, efficient and scalable.” On replacing data cleansing services and software: “No, it will not. AI will enable data cleansing at scale. The amount of time we spend cleaning data is a huge expense. If we can get AI to clean huge amounts of data, it will make our downstream systems and reports far more efficient.”
--Jack Koczela, director, Sourcing & Transformation, Supply Chain, Froedtert Health
“[AI] can use more information that may not be considered today including unstructured information such as sound and image.”
--Joe Dudas, division chair, Supply Chain Innovation and Strategy, Mayo Clinic
“By surveying many sources of data available and ensuring the comprehensiveness and accuracy of product information, including UDI data.”
--Arnold Chazal, CEO, VUEMED Inc.
“Something AI could potentially be used for is cleaning and normalizing asset lists to understand the make and model of equipment in use by the provider. This could lead to improvement in inventory tracking and management of supplies and resources and a more streamlined procurement process by having more information to identify the best suppliers and parts.
“Also, one method our teams at PartsSource are working on integrating AI is through the comparison of vendor agreement terms and conditions that can also be normalized in the pricing process. By doing so, PartsSource members that have access to our Repair and Service Agreements solution will be able to increase efficiency by using an automated process to compare vendors and consolidate them into one contract at one price. This in turn, increases not only cost savings and efficiency, but also capacity for healthcare technology management and clinical engineering teams by freeing up time and resources that would normally be used to compare and manage vendor contracts.”
--James Kollai, senior director, Client Solutions, PartsSource
“AI can assist in providing an auditing function for many of the underlying datasets that exist within the healthcare supply chain. For example, if the product unit price was added incorrectly and/or the unit of measure doesn’t match similar items then there are opportunities to proactively address data integrity concerns to eliminate the potential of downstream rework with order processing and accounts payable. Additionally, there may be opportunities to identify duplicate data and anomalies, product naming nomenclature standardization and audit data files for incomplete and/or inconsistent information, to name a few.”
--Tom Redding, senior managing director, Healthcare, St. Onge Co.
“AI could review contract activation data at the item level ,– review price and unit of measurement information versus benchmark data and generate an error message if the data is out of line.”
--George S. Godfrey, Chief Supply Chain Officer and corporate vice president, Financial Shared Services, Baptist Health South Florida
“Data integrity and data completeness and usefulness is one of the primary areas for AI to benefit the healthcare supply chain. We use AI to authenticate unit of measure data from customer files, find and remove out-of-tolerance prices, sort out mismatches between purchase unit of measure requests and price. For example, did the customer really buy a case or box of a certain product? Also, AI can be used to ensure data is properly synced between disparate operating systems like the ERP and the EHR or other supply chain tools like the inventory systems and the ERP.”
--Carl Natenstedt, CEO, Z5 Inventory
2. To adopt and implement supply data standards and escalate UDI application
“AI can automate the exchange of supply data between different systems and stakeholders. This can streamline the UDI compliance process and ensure that all parties have access to the necessary information.”
--John Freund, Jump Technologies
[AI] can find the not so obvious patterns and can also provide information in context (as it is needed).”
--Joe Dudas, Mayo Clinic
“By expanding the use of standards such as UDI, filling the data gaps and ensuring the dissemination of accurate data across hospitals IT systems cohesively and in real time.”
--Arnold Chazal, VUEMED
“FDA mandates that medical/surgical equipment obtain proper clearances before being utilized in healthcare practices. As such, manufacturers need to abide by UDI standardizations. However, in the real world, different standards, like GS1, HIBCC or UNSPEC, are adopted by various manufacturers. AI can help by lowering the impedance mismatch among various standards. AI-built and managed equivalency knowledge representations will help with proper device identification, tracking, inventory control and recall management.
“Having the proper device identity is critical to any tracking and recall management, especially if the item is serial or lot controlled. This is where universality and uniqueness come into the picture. Where AI comes in is through a decision support system, where it can facilitate the identification of all the relevant, related and affected items and their distribution across the health care provider network.”
--Vasco Kollokian, Tecsys
“AI can help through the development of compliance screenings to determine where gaps exist in UDI implementation. By doing so, it can increase supply chain visibility by identifying inaccurate or incomplete data. It can also assist in driving strategic decision-making by providing key information that could benefit sourcing strategies and other data-driven decisions. AI can also be used to streamline procurement. For instance, AI can determine where items are the same and establish standardized vendor procurement to maximize cost based on purchasing power.”
--James Kollai, PartsSource
“Adoption of data standards and solutions, like UDI, HL7, and FHIR make data more streamlined and enable for the safer sharing of sensitive data. UDI enables the tracing of data back to its provenance to ensure it is pure and has not been tampered with. AI can be of help here, for example, by:
- Helping to convert non-standard data to data compliant with data standards.
- Working with UDI solutions to identify, track, and trace medical devices and data.
- Dynamically analyzing standardized data to identify new and emerging threats.”
--Bruce Lieberthal, vice president and Chief Innovation Officer, Henry Schein
“Using AI to implement data standards could be achieved through use of Natural Language Processing and a rule-based approach to create the necessary data structure. Without using AI, the process of implementing data standards can be time consuming and challenging to maintain. When considering how many different people are making changes daily to the ERP system and/or other subsystems, there is a need to have a reliable method to monitor and adjust data. Health systems can’t continue the current process of cleansing their data is a quarterly and/or semi-annual basis and keep up with all the data requirements.”
--Tom Redding, St. Onge Co.
“AI may assist in escalating UDI application or adoption, but not only through gathering standardized data. Rather, I think providers will see the value of broad UDI adoption as a tool to provide the better data quality needed for large AI models.”
--Jack Koczela, Froedtert Health
3. To integrate computer systems (e.g., with accounting and billing, EHR/EMR, manufacturer/distributor ordering systems and/or device and equipment technologies internally and with suppliers for service
“Among other things, AI can be used to standardize data from disparate sources, map and match data elements from different systems, cleanse and validate data, and integrate data from different systems into a single repository or federated database.”
--John Freund, Jump Technologies
“Modern interfaces (API) are built on standards that are a lot easier to implement. APIs allow real-time transactions that can speed up the supply chain as well as make it much smarter.”
--Joe Dudas, Mayo Clinic
“By being able to set and maintain data standards across internal and external systems to ensure better communication and facilitate process integration upstream in the supply chain and downstream in the clinical areas with clinical data.”
--Arnold Chazal, VUEMED
“Integrating multiple computer systems that deal with various aspects of supply chain in healthcare, ranging from accounting to electronic medical records to supplier portals, is challenging not because of the technology’s complexity. The real issue lies in the human reluctance to embrace such integration. While AI can fill in some gaps and facilitate data standardization and exchange, the underlying problem can often be attributed to competitiveness among organizations, which suffer from the ‘’Not-Invented-Here Syndrome” which resist using ideas from outside their own walls.
“If AI has to access historical ordered items and their quantities, and has real-time visibility into levels of inventory and their shelf life, is able to calculate consumption rates at various point-of-use locations, then in principle it can efficiently combine these multi-dimensional sources of information to automatically procure what is needed, when and in what quantity, controlling levels of replenishments where they are needed at the right time and cost, avoiding unnecessary expiration-driven waste.
“The adoption of AI [requires] trust. While these systems may be a certain level of maturity (or lack there-of), humans typically would like to be in control, let AI suggest and humans act. Which characterizes AI in this case as ‘Augmented Intelligence’, akin to GitHub copilot for developers from Microsoft. It also depends on whether the AI is simply ordering an incorrect amount of inventory versus delivering an ionizing dose of radiation to a patient – different consequences.
“I would characterize the role of AI – especially in this case- as ‘glue’ that comes on as a set of components, disjointedly under the control of potentially competing vendors. The overarching end-of-end value promised by AI in a domain such a supply chain, partially controlled by more than one vendor, seems to remain – at least in the near term – relatively fractured. However, as seamless access to data directly (through API) or otherwise (through extrapolation and third-party sources) becomes more prevalent, the fractured nature of AI—based value will undoubtedly coalesce, albeit at a slow pace.
--Vasco Kollokian, Tecsys
“The normalization of the data across platforms is one way the integration of AI with computer systems can be beneficial. AI can help in identifying duplicate patients, potential insurance fraud, revenue opportunities, etc. as the data becomes cleaner and more integrated across platforms. This enables pattern recognition algorithms for Deep Learning to be utilized for issues and opportunities. Also, with system integration, there will be a corresponding productivity benefit for the patients and providers as scheduling improves, spend is more visible, and staff can be better utilized.”
--James Kollai, PartsSource
“AI can automate data entry, extraction, and use in health care information systems. AI is used to harmonize data across various data formats and systems and can help healthcare systems safely share data. AI can identify and fix data errors. AI can assist in data localization from one language/geography to another. AI may be used for clinical decision support solutions that assist providers in making faster, more accurate diagnoses and therapeutic decisions. AI automates patient engagement and safe access for patients to their health care data. AI may also help patients make better decisions as to what the data means, how to act, and who to speak with.”
Bruce Lieberthal, Henry Schein
“AI is critical to ‘connect the dots’ between all the various systems and proactively identify data gaps and resolve independently to ensure a healthy supply chain. The current approach of waiting until an error occurs then addressing the error doesn’t resolve the underlying reason for the error in the first place. AI will become the ‘quarterback’ for all the various internal and external systems to be the play caller on where best to apply the necessary resources to streamline the operation.”
--Tom Redding, St. Onge Co.
“I think this type of application will more often come from a third party such as ChatGPT or other large language model (LLM) solutions. If providers adopt an LLM solution, I believe that they will be able to gain incredible efficiencies through allowing artificial intelligence to sift through the mountains of data that we already have captured in our many, but disconnected, systems. I think that AI deployed through [robotic process automation] can help link systems together. It is more efficient for the [software-as-a-service] vendors to develop a prebuilt/linked system at scale, rather than each of us developing one off solutions.”
--Jack Koczela, Froedtert Health
“AI could link to our recall system, generate messages to internal clinical departments and review contractual commitments that are missed by manufacturers.”
--George S. Godfrey, Baptist Health South Florida
“AI is best used to analyze and correct cross platform data inconsistencies. AI can identify the mismatched transactions and suggest possible corrections for human review. It can also be used to prevent future mistakes by identifying corrections to previous mismatched transactions and fixing the source data causing repeat mistakes. In this way, AI can help humans to improve their systems and generate increased efficiency in their supply chain teams.”
--Carl Natenstedt, Z5 Inventory
GHX offers quick tutorial on AI-supply chain links
Archie Mayani, Chief Product Officer, GHX, appreciates how artificial intelligence can assist healthcare supply chain performance improvement as well as how supply chain executives and professionals show growing interest in the technology. But she recommends that the industry takes this technology extremely seriously. Here’s why.
Building Generative Artificial Intelligence (GenAI) and [Machine Learning]/AI healthcare applications involves handling sensitive data, and implementing robust data governance practices is crucial. Here are some best practices for data and governance in the development of GenAI and AI healthcare applications:
- Data Privacy and Security: Implement encryption and other security measures to protect genomic and healthcare data. Adhere to industry standards and regulatory requirements (e.g., HIPAA, GDPR, UDI) for data privacy.
- Data De-identification Techniques: Implement robust data de-identification techniques, such as anonymization or pseudonymization, to protect patient privacy while still allowing for meaningful analysis and application functionality.
- Informed Consent, Data rights and Ethical Considerations: Clearly communicate data usage policies to patients and obtain informed consent for customer data collection. Adhering to ethical guidelines to ensure responsible and transparent use of data with appropriate data rights is critical.
- Interoperability Data Quality and Standardization: Implement data validation processes for high-quality healthcare data and standard data formats and coding systems for consistency and interoperability. Design applications to be interoperable with existing healthcare systems and databases. Utilize standards like EDI, GTIN, FHIR (Fast Healthcare Interoperability Resources) for seamless data exchange.
- Data Governance Framework: Establish a comprehensive data governance framework that includes policies, procedures, and guidelines for data management, usage and compliance. Regularly review and update these policies to adapt to changing regulations.
- Transparent Explainability: This is most critical! Ensure transparency and explainability of AI algorithms. Provide clear documentation on how algorithms operate and make decisions, especially in applications involving patient care.
- Continuous Monitoring and Compliance: Implement continuous monitoring mechanisms to help ensure ongoing compliance with data protection regulations. Regularly conduct risk assessments and audits to identify and address potential vulnerabilities.
- Collaboration and Stakeholder Involvement: Collaborate with relevant stakeholders, including healthcare providers, researchers and regulatory bodies. Involving stakeholders helps ensure that the application aligns with industry standards and addresses specific needs.
By integrating these best practices into the development and deployment of GenAI and AI healthcare applications, developers can ensure the responsible and ethical use of genomic and healthcare data, fostering trust among patients, healthcare professionals and regulatory bodies.
Healthcare is still early in its journey of leveraging AI to transform the supply chain. AI will help providers in a multitude of ways moving forward; however, it is still important for organizations to tread carefully in these new waters. While providers will consider how and why they should implement AI, it’s important they identify a trusted resource as they embark on this innovation journey. Technology partners have an opportunity to act as an enabler for providers implementing AI, leveraging the right technologies to solve customer problems, whether that is to reduce clinical burnout or driving overall value-based care.
AI and healthcare: A courtship of convenience, convergence
For better or for worse, artificial intelligence and all its ilk, including generative and machine-learning versions, have captivated the hearts, interests and minds of consumers and industries everywhere – healthcare being no exception.
From a content perspective, educators fear (but students love) its ability to craft essays and research papers; Hollywood screenwriters despise (but studios secretly favor) its ability to pen scripts; media outlets quiver about promoting AI-created photorealistic fake images and videos and issue codes of ethics as guidelines for use; corporate executives appreciate its ability to collect and crunch data for analysis even as they establish usage guardrails for employees; and healthcare organizations espy a litany of clinical, financial and operational benefits – from providers to payers to suppliers – even as they worry about burgeoning cybersecurity threats.
During the last three years, the global AI industry reached $240 billion in value and 250 million users worldwide, according to Altindex.com, which also projects the global AI market to exceed $500 billion in value by 2027.
Based on the quantity of media releases that Healthcare Purchasing News has received during the last year alone, healthcare seems quite enamored with the promise that AI brings. A curious headline from the public relations segment of the media industry speaks volumes: “Made by Humans: The New Gold Standard for Marketing and PR Output?”
What follows are a sampling of curated headlines and media release promotions with live links trumpeting noteworthy applications, some delicately seasoned with a pinch of hyperbole.
“Getting technical with US consumers on healthcare,” https://7796197.fs1.hubspotusercontent-na1.net/hubfs/7796197/GRAPHICS/23_CAR011_Consumer_Survey_Infographic_F%20%281%29.pdf
“AI is revolutionizing the visualization of heart conditions, enabling doctors to predict the likelihood of a heart attack or the development of cardiovascular disease like never before.”
“AI technology is playing an increasingly prominent role in clinical trials in the U.S., with large biotech and pharma companies training AI to find suitable trial patients.
“To increase patient participation diversity, especially with African American participation in clinical trials as low as 4.8%, drug giants are looking toward AI for success.”
“To streamline cardiac monitoring and virtual telemetry, InfoBionic, a digital health company providing AI-powered diagnostic remote patient monitoring solutions, recently announced its collaboration with Mayo Clinic to incorporate its extensive know-how in cardiac patient monitoring, AI-ECG, and virtual telemetry to optimize and build upon its existing monitoring platform.”
“IBM Study: Businesses Work on Adapting to Generative AI, Hybrid Cloud,” https://www.techrepublic.com/article/ibm-hybrid-cloud-user-survey/?utm_source=Sailthru&utm_medium=email&utm_campaign=e312_11.21.23_DT_Update_Paylocity_Spotlight&utm_term=daily-tech-insider-active
“Premier Weighs in with FDA on Use of AI in Drug Manufacturing,” https://premierinc.com/newsroom/policy/premier-weighs-in-with-fda-on-ai-in-drug-manufacturing
“AI is helping translate disparate medical records.”
“Avoiding the GenAI hype machine,” https://www.oliverwyman.com/our-expertise/perspectives/health/2023/november/avoiding-the-gen-ai-hype-machine.html?utm_source=dynamics-marketing&utm_medium=email&utm_campaign=owhealth-newsletter&utm_content=17-nov-2023&utm_id=cmp-15851-j9l8k4#msdynttrid=OQshi39mSGBlQBf2_6KRrA9CwrOtUdAXoL8qQ7KUF8g
“Free AI health assistant app that allows you to scan your face and eyes and measure vitals with your phone camera.”
“The potential power of AI in bridging the gap between our lifespan and healthspan.”
“FDA Launches Pilot Program to Help Further Accelerate Development of Rare Disease Therapies,” https://www.fda.gov/news-events/press-announcements/fda-launches-pilot-program-help-further-accelerate-development-rare-disease-therapies
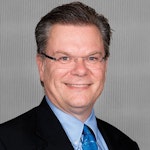
Rick Dana Barlow | Senior Editor
Rick Dana Barlow is Senior Editor for Healthcare Purchasing News, an Endeavor Business Media publication. He can be reached at [email protected].